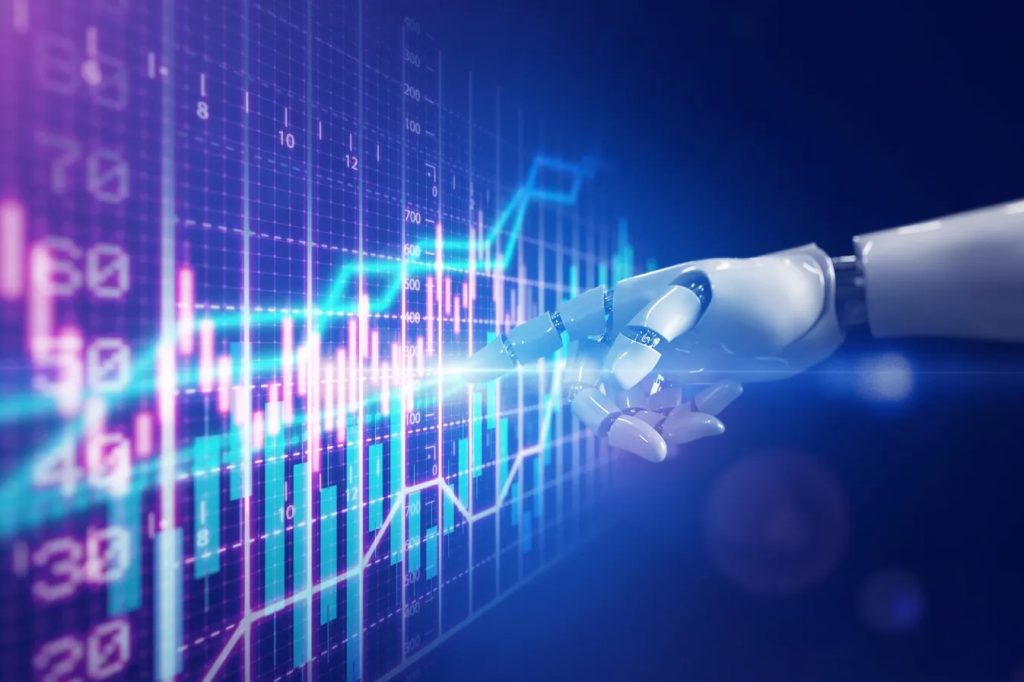
In the ever-evolving landscape of financial markets, the symbiotic relationship between artificial intelligence (AI) and machine learning (ML) has become increasingly pronounced. These technological advancements are reshaping stock analysis, transcending traditional methods and introducing new paradigms. In this discourse, we delve into the profound impact of AI and ML on stock analysis, examining both their applications and the challenges they pose.
Applications of AI and ML in Stock Analysis
- Algorithmic Trading: Precision in Motion
- Algorithmic trading, powered by AI, automates buy and sell decisions based on predefined rules. These algorithms analyze historical data, market indicators, and real-time information to execute trades swiftly and efficiently.
- By removing emotional biases and responding to market fluctuations in milliseconds, algorithmic trading enhances liquidity and contributes to overall market efficiency.
- Risk Management: Navigating the Uncertainty Seas
- ML models excel at risk assessment. They analyze historical volatility, correlations, and macroeconomic factors to quantify risk exposure.
- Portfolio managers rely on these models to optimize risk-return trade-offs, ensuring prudent investment decisions.
- Sentiment Analysis: Decoding Market Emotions
- Natural language processing (NLP) techniques enable sentiment analysis. By analyzing news articles, social media posts, and corporate communications, AI gauges market sentiment.
- Understanding investor sentiment provides valuable insights for predicting market movements.
Challenges and Considerations
- Data Privacy and Security
- Handling sensitive financial data demands robust security measures. Encryption, access controls, and compliance with data protection regulations are imperative.
- Balancing data accessibility with privacy protection remains an ongoing challenge.
- Regulatory Compliance
- Financial institutions must navigate a complex web of regulations when implementing AI solutions. Compliance ensures ethical use and prevents unintended consequences.
- Striking the right balance between innovation and adherence to legal frameworks is crucial.
- Ethical Dimensions
- Fairness, transparency, and bias mitigation are ethical imperatives. AI models must be designed to avoid discriminatory outcomes.
- Responsible AI practitioners actively address biases and promote fairness.
Benefits and Future Prospects
- Efficiency and Accuracy
- AI processes vast datasets swiftly, enabling faster decision-making.
- ML models learn from historical data, potentially improving accuracy in stock price predictions.
- Adaptability
- AI systems adapt to changing market conditions, a critical trait in dynamic financial landscapes.
- As AI algorithms evolve, their adaptability will continue to enhance stock analysis.
- Cost Savings and Scalability
- Automation reduces operational costs, making AI-driven stock analysis economically viable.
- Scalability allows AI models to handle increasing data volumes without compromising performance.
Conclusion
The impact of AI and Machine Learning on stock analysis is undeniable. As we peer into the horizon, collaboration among researchers, financial institutions, and policymakers will shape the future. Responsible adoption, ethical considerations, and continuous innovation will determine whether these technological marvels remain allies or become unintended adversaries in our financial saga.