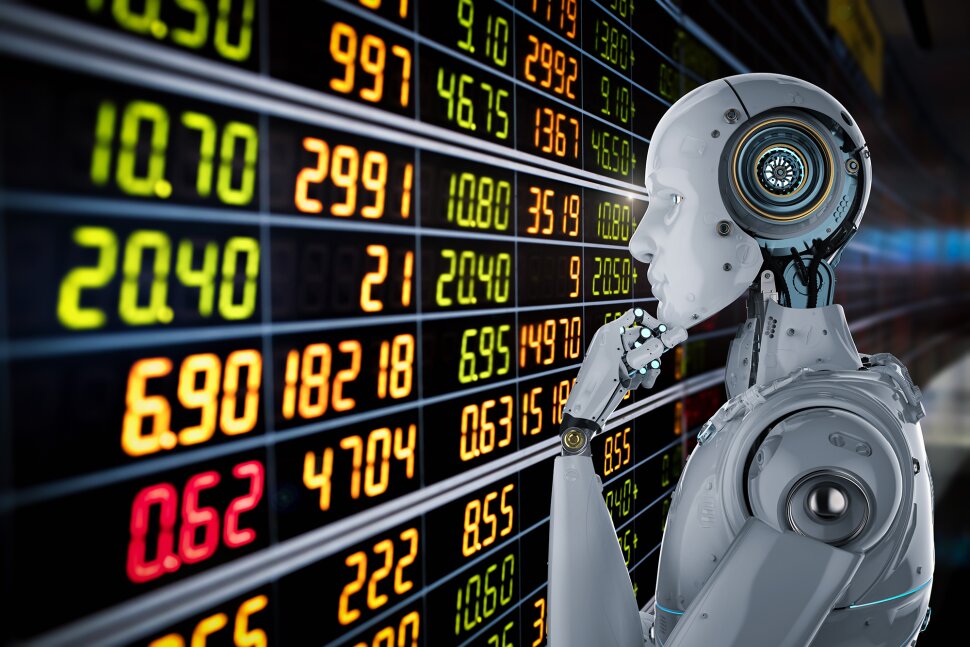
Using big data and machine learning to identify value investment opportunities is a fascinating topic that combines cutting-edge technology with the timeless principles of value investing. Let’s dive into it.
1. The Intersection of Finance and Technology
In recent years, the financial industry has witnessed a seismic shift. The convergence of big data, artificial intelligence (AI), and machine learning (ML) has opened up new avenues for investors. Let’s explore how these technologies are transforming investment strategies:
2. Data-Driven Insights
- Big Data: The sheer volume of financial data available today is mind-boggling. From stock prices and economic indicators to social media sentiment and satellite imagery, there’s an ocean of information. Big data allows us to harness this wealth of data and extract meaningful insights.
- Machine Learning: ML algorithms can sift through vast datasets, identifying patterns and relationships that humans might miss. These algorithms learn from historical data and adapt over time, improving their predictive accuracy.
3. Value Investing and ML
- Traditional Value Investing: Value investing, popularized by legendary investors like Benjamin Graham and Warren Buffett, focuses on buying undervalued stocks. It involves analyzing fundamentals (such as price-to-earnings ratios, book values, and dividends) to find companies trading below their intrinsic value.
- Enhancing Value Strategies with ML:
- Quantitative Models: ML models can enhance traditional value strategies. For instance, they can identify undervalued stocks based on a broader set of criteria, including non-traditional data sources.
- Factor-Based Investing: Factors like low volatility, quality, and momentum play a crucial role in stock returns. ML models can dynamically adjust factor weights, optimizing portfolio performance.
- Sentiment Analysis: ML algorithms analyze news articles, social media posts, and earnings calls to gauge market sentiment. Positive sentiment might indicate undervaluation.
- Predictive Models: ML can predict future stock prices, helping investors make informed decisions.
4. Challenges and Considerations
- Data Quality: Garbage in, garbage out. High-quality data is essential for ML models. Cleaning and preprocessing data is a critical step.
- Overfitting: ML models can overfit historical data, leading to poor out-of-sample performance. Regularization techniques mitigate this risk.
- Interpretability: Some ML models (like deep neural networks) lack transparency. Explainable AI methods are gaining traction.
- Ethical Concerns: Bias in ML models can perpetuate inequalities. Ensuring fairness and avoiding discrimination is crucial.
5. Policy Implications
- Policymakers need to strike a balance between fostering innovation and safeguarding against risks.
- Regulations should encourage responsible AI adoption while addressing potential pitfalls.
Remember, investing is both an art and a science. While ML can enhance decision-making, it doesn’t replace human judgment. Successful investors combine data-driven insights with a deep understanding of business fundamentals.